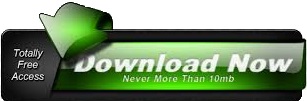
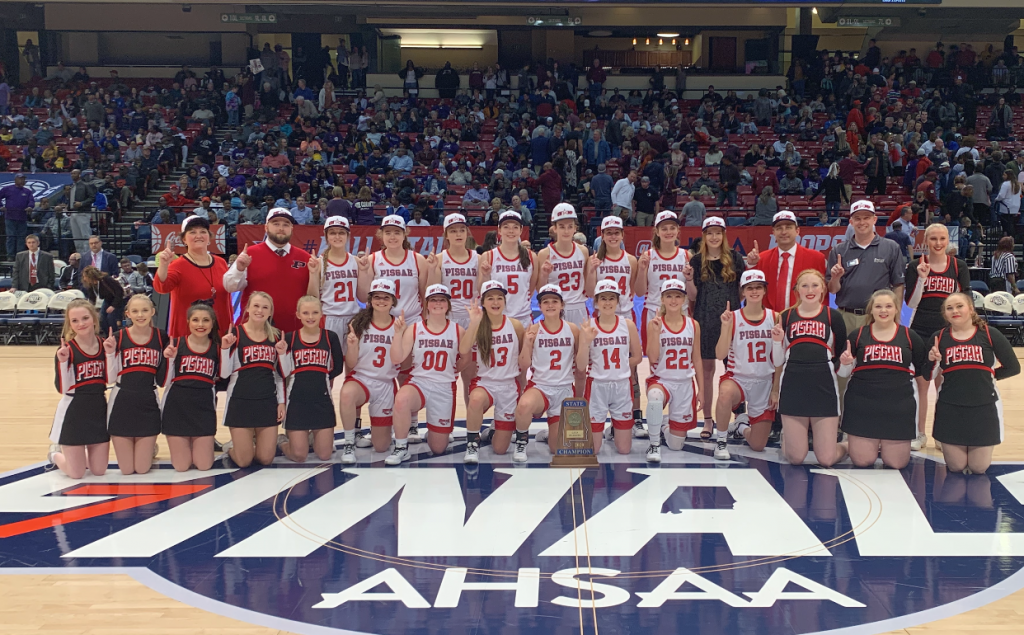
Reorder to (0,1,2) # Third "=>" Change to 3 and 2 In exchange for, Corresponding to the parameters in the function 0 Immobility ,1 and 2 In exchange for, After the transformation, it is (0,2,1), The corresponding dimension is (5,2,3). Reorder to (0,1,2) # the second "=>" Change to 3 and 5 In exchange for, Corresponding to the parameters in the function 2 Immobility ,0 and 1 In exchange for, After the transformation, it is (1,0,2), The corresponding dimension is (5,3,2). Parameter transformation in function ( 0, 1, 2 ) = > ( 0, 2, 1 ) = > ( 1, 0, 2 ) = > ( 0, 2, 1 ) # first "=>" Change to 2 and 5 In exchange for, Corresponding to the parameters in the function 0 Immobility ,1 and 2 In exchange for, After the transformation, it is (0,2,1), The corresponding dimension is (3,5,2). In fact, the two parameter exchange includes the following partsĭimension transformation focus Dimensional numerical transformation : ( 3, 2, 5 ) = > ( 3, 5, 2 ) = > ( 5, 3, 2 ) = > ( 5, 2, 3 ) # The reader must consider what the next line stands for Intuitively, it is to put 0 and 2 The two positions exchange directly Split line - Explanation partįour changes permute Parameter put (0,1,2) In exchange for (2,1,0) Parameters 2 Corresponding 5 The column has become 3 Column Now the parameters 0 Corresponding 3 Block pass permute Has become 5 block permute ( 2, 1, 0 ) # Swap blocks and columns print (b ) print (b. Split line -Ĭhange 4 :0 And 2 In exchange for b = x. Here take the classic change 4 as an example If you have energy, you can read this article, Be able to better understand the dimensional change process of functionĪnd deepen the impression of the output results Two 、 Explain with examples This article is this one torch in permute() The usage function The supplementary content of the article Thank you very much More details are added to the case in this paper Then I'll talk about it in detail permute The detailed process of dimension change in function So in order to sum over it we have to collapse its 3 elements over one another: > torch.I wrote a piece before torch in permute() The usage function article, Unexpectedly, there are many collections and likes The first dimension ( dim=0) of this 3D tensor is the highest one and contains 3 two-dimensional tensors. That’s why I think some basic visualizations of the process of summation over different dimensions will greatly contribute to a better understanding. When we look at the shape of a 3D tensor we’ll notice that the new dimension gets prepended and takes the first position (in bold below) i.e. However, it becomes trickier when we introduce a third dimension. She explains very well the functioning of the axis parameter on numpy.sum. So when it collapses the axis 0 (the row), it becomes just one row (it sums column-wise). The way to understand the “ axis” of numpy sum is that it collapses the specified axis. The key to grasp how dim in PyTorch and axis in NumPy work was this paragraph from Aerin’s article: Numpy.sum(a, axis=None, dtype=None, out=None, keepdims=False) NumPy sum is almost identical to what we have in PyTorch except that dim in PyTorch is called axis in NumPy: So why is that? I found out an article of Aerin Kim 🙏 tackling down the same confusion but for NumPy matrices where we pass a second parameter called axis. I was surprised to see that the reality was the opposite of what I’ve expected because I finally got the result tensor but when passing the parameter dim=1: > torch.sum(x, dim=1) tensor() But it turned out I got something different: a 1x3 tensor. Following the reasoning that the dimension dim=0 means row-wise, I expected torch.sum(x, dim=0) to result in a 1x2 tensor ( 1 + 2 + 3 and 4 + 5 + 6 for an outcome of tensor). We specify at first the rows (2 rows) and then the columns (3 columns), right? That brought me to the conclusion that the first dimension ( dim=0) stays for rows and the second one ( dim=1) for columns.

So for a 2x3 tensor we’ve 2 rows and 3 columns: > x = torch.tensor(, ]) > x.shape torch.Size() When we describe the shape of a 2D tensor, we say that it contains some rows and some columns. However, the more important problem was, as I said, the direction of each dimension.
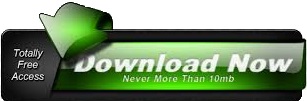